Classification and Biomass Evaluation in Optical Images
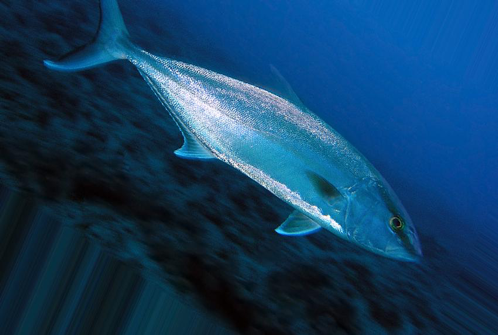
Description of work
In this WP, we will develop novel image segmentation and classification techniques to identify the type of the detected pelagic fish from optical underwater images. To face the challenge of the changing water environment, and the expected diversity of the detected fish, our solution will be based on blind classification while fusing prior knowledge on the characteristics of the tracked pelagic fish, namely, their motion, size, texture, and colour. Besides classification, we will develop an algorithm to evaluate the biomass of detected fish.
The tasks identified with this WP are:
3.1) Segmentation of Optical Images: Lead partner - UH. Design an algorithm to segment an underwater optical image. The developed algorithm will utilize the expected correlation between the pixels of the image by adding an intermediate soft classification layer within the classical model-based segmentation process. During this task, we will also extract the size, texture, and shape features of all objects identified as ”fish” from the segmented image. The segmented image and the extracted object features will serve as an input in Task 3.2 (optical classification). The work will be managed by UH, and will involve EvoLogics to learn of the expected noise characteristics in the optical image, and to practice on a database of underwater optical images. The milestone of this WP is the comparison of ground truth labelling with detection decisions based on the segmented images.
3.2) Optical Classification: Lead partner – IMDEA. Development of blind machine learning-based classification to identify if the detected fish belongs to the set of tracked pelagic fish. The classification algorithm will fuse prior information of the characterization of the tracked pelagic fish, and will combine information gathered during the process of acoustic classification. The classification output will be sent to shore (WP4). The work will be managed by IMDEA, and will involve UH for fusing the acoustic classification data and the prior data of the fish characterization. The milestone of this WP is the comparison of ground truth labelling with identification decisions of fish.
3.3) Optical Quantification of Fish Biomass: Lead partner – PT. Design of an innovative tool to estimate the size and the number of detected fish in an optical image using deep-learning. The tool will involve the setup of an unsupervised neural network that will learn to accurately estimate the total number of objects detected in a segmented image, along with their overall biomass. Together with the classification output obtained in Task 3.2, the fish count and biomass evaluation will be sent to shore (WP4). The work will be managed by PT, and will involve IMDEA and UH for fusing the optical image segmentation and classification. The milestone of this WP is the comparison of ground truth quantification of fish with net catches.